Revolutionizing Business with Machine Learning Labeling Services
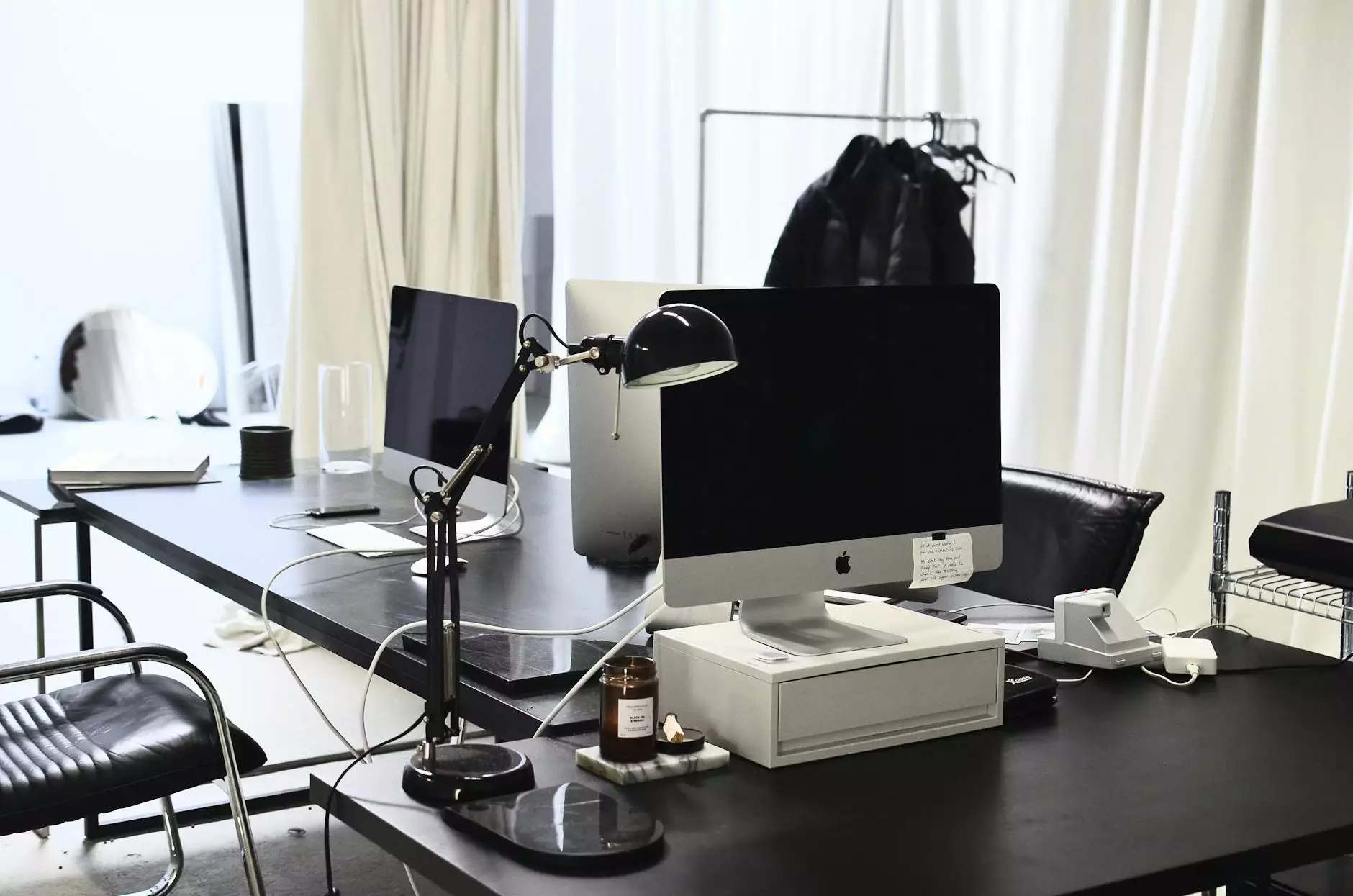
In today’s rapidly evolving digital landscape, businesses are continually seeking innovative solutions to enhance their operations, streamline processes, and provide exceptional services. One of the groundbreaking technologies that have emerged as a game-changer in various industries is machine learning. Specifically, machine learning labeling services are playing a crucial role in helping organizations leverage data more effectively. This article delves into the impact of these services on business, exploring their benefits, applications, and relevance in the modern economy.
Understanding Machine Learning Labeling Services
Machine learning labeling services involve the process of annotating data—assigning labels to data points which can then be used to train machine learning algorithms. This is an essential step in developing accurate and reliable AI models, as the quality of labeled data directly influences the effectiveness of the machine learning process.
Why is Data Labeling Critical?
Data is often referred to as the new oil, and just like crude oil, it must be refined to be useful. In the context of machine learning, labeling data is akin to refining oil; it transforms raw data into a structured format that algorithms can understand. Without properly labeled data, machine learning models cannot produce the desired outcomes.
The Benefits of Machine Learning Labeling Services
Integrating machine learning labeling services into business operations can lead to numerous benefits, including:
- Enhanced Accuracy: Properly labeled data allows machine learning models to achieve higher accuracy in predictions and classifications, which is critical in sectors like healthcare, finance, and autonomous vehicles.
- Increased Efficiency: Automating the data labeling process can significantly reduce the time and effort required to prepare datasets, allowing businesses to deploy machine learning solutions faster.
- Cost-Effectiveness: Outsourcing labeling services can be more cost-effective than in-house solutions, particularly for organizations that require large volumes of data to be labeled quickly.
- Scalability: As businesses grow, so does their need for data labeling. Utilizing labeling services makes it easier to scale operations according to demand.
- Access to Expertise: Professional labeling services often employ skilled annotators who are experienced in various domains, ensuring high-quality data labeling.
Applications of Machine Learning Labeling Services
The applications of machine learning labeling services span across numerous industries:
1. Healthcare
In the healthcare industry, accurate data labeling is crucial for developing models that can predict diseases, analyze medical images, and enhance patient care. For instance, labeling radiology images helps in training algorithms to detect anomalies such as tumors or fractures.
2. Autonomous Vehicles
Autonomous vehicles rely heavily on machine learning to interpret their surroundings. Accurate labeling of road signs, pedestrians, and other vehicles is paramount for safe navigation. Labeling services contribute to creating datasets necessary for training these complex systems.
3. E-commerce
E-commerce platforms leverage machine learning for personalized recommendations, customer segmentation, and sentiment analysis. Product image labeling and categorization enhance the shopping experience by ensuring that users receive relevant suggestions.
4. Finance
In finance, machine learning is utilized for credit scoring, fraud detection, and risk assessment. Labeling historical transaction data helps in training models to identify patterns indicative of fraudulent behavior, safeguarding both businesses and customers.
Choosing the Right Machine Learning Labeling Service
When selecting a machine learning labeling service, consider the following factors:
- Quality Assurance: Ensure that the service provider has stringent quality control measures in place to maintain high labeling standards.
- Experience and Expertise: Look for providers with experience in your industry and knowledge of specific data types.
- Customization: Choose a service that offers tailored solutions to meet your unique data requirements.
- Scalability: Opt for a provider that can handle varying volumes of data as your business grows.
- Technology Compatibility: Ensure the labeling service integrates seamlessly with your existing systems and workflows.
Implementing Machine Learning Labeling Services
Successfully implementing machine learning labeling services requires a strategic approach:
1. Define Your Objectives
Start by clearly defining the objectives of your machine learning project. Understanding the problems you want to solve will help you determine the type of data labeling needed.
2. Select the Right Data
Identify the datasets that require labeling. Ensure the data is representative, diverse, and of high quality, as this will directly influence the performance of your machine learning models.
3. Partner with a Reliable Service Provider
Choosing a reputable labeling service provider is crucial. Research their track record, read reviews, and request samples of their work to gauge their expertise.
4. Monitor and Evaluate
After implementing the labeling service, continually monitor the output for quality and accuracy. Regular evaluations will help you maintain high standards and make necessary adjustments to improve the process.
The Future of Machine Learning Labeling Services
The future of machine learning labeling services looks promising. As technology continues to evolve and data generation increases, the demand for high-quality labeled data will only grow. Innovations such as semi-supervised learning, active learning, and automated labeling are expected to shape the industry, making data labeling even more efficient and accessible.
Challenges and Considerations
While the advancements in machine learning labeling services are exciting, several challenges remain:
- Data Privacy: With increasing regulations around data privacy, ensuring compliance while labeling sensitive data is essential.
- Bias in Data: It’s critical to address biases in training data to avoid perpetuating them in machine learning models, which can lead to skewed results.
- Resource Allocation: Finding the right balance between internal resources and outsourcing labeled services can be complex.
Conclusion
The integration of machine learning labeling services into business operations presents a unique opportunity to harness the power of artificial intelligence. By ensuring that data is accurately labeled, organizations can develop effective machine learning models capable of transforming operations and enhancing customer experiences. As the digital landscape continues to evolve, the importance of high-quality labeled data cannot be overstated. By embracing these services, businesses can position themselves at the forefront of innovation, driving success and growth in the years ahead.